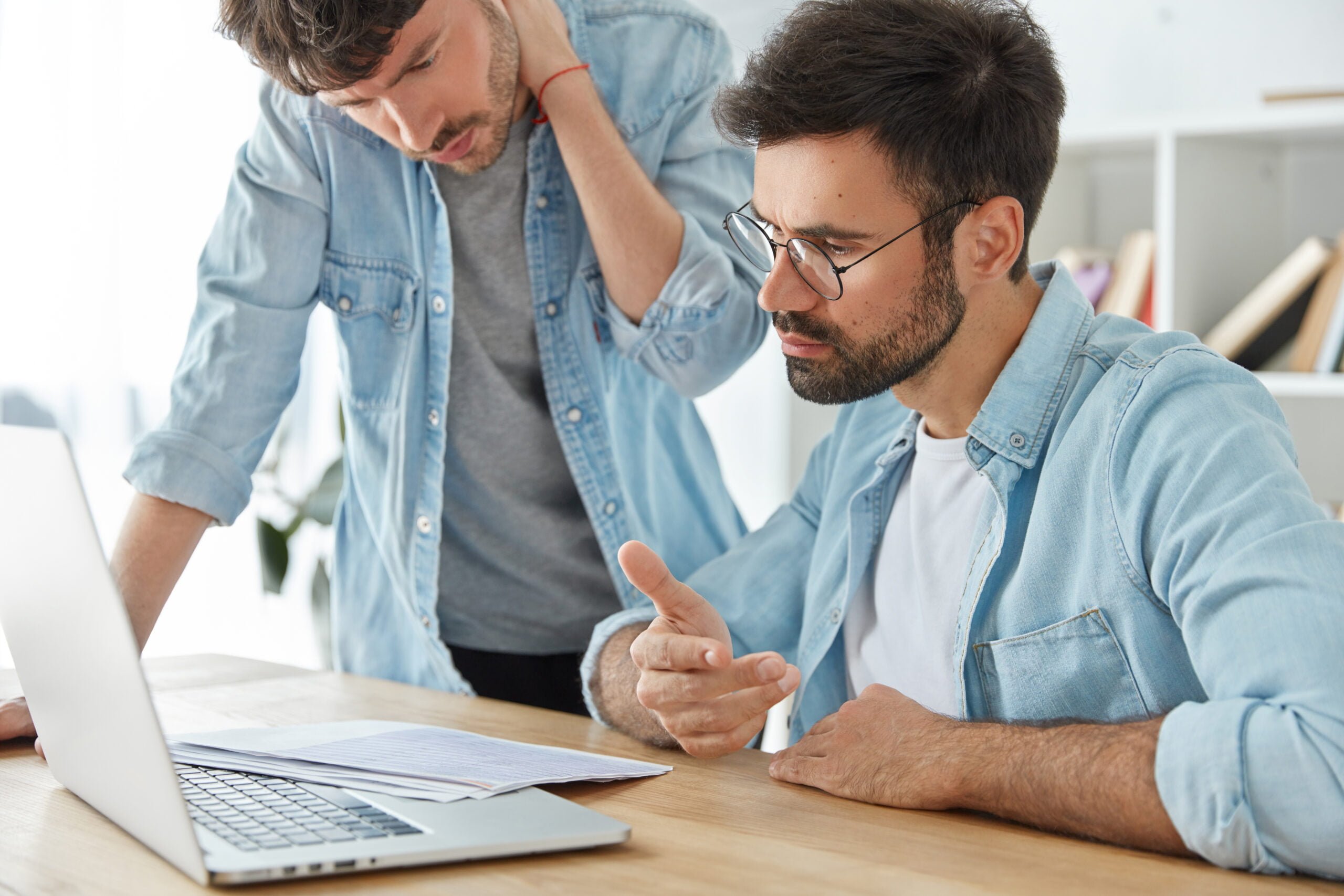
Anyone who spends some time in marketing quickly learns that its efficacy largely comes down to one factor – experimentation. Even if the business community lauds a new feature or names a new “marketing hack that will change your company”, you’ll have to put it to the test to check if it actually works for your organization. Plus, you won’t be able to secure buy-in for a new marketing initiative or change from the organization without proof of concept – so, in this case, showing numbers that prove that the tactic works.
But how do you get the data? Here’s where A/B testing comes into play.
In this article, we’ll discuss how to conduct A/B testing and what the benefits of using it to verify your marketing campaigns are. But first, let’s start with an A/B testing definition.
What is A/B Testing in Marketing
A/B testing is a research method that allows for comparing two different versions of a digital asset such as ads, webpages, emails, marketing copy, etc. to identify the best-performing one. Version A is shown to one group of people, and version B to another. After analyzing the results, marketers can determine which version is more effective at achieving a specific business goal, for example, driving more ROI.
Typical use of A/B testing involves enhancing UI/UX, optimizing marketing campaigns, and improving conversions.
A/B Testing Examples
So, what is A/B testing used for? To give you a sense of what it can reveal, here are two A/B test examples – one for paid ads in search, and one for email marketing.
Scenario – Google Ads
Let’s say that you run an e-commerce store selling yoga and pilates clothing. Your goal is to improve CTR from your PPC campaigns, and you want to check if adding an incentive in the copy will do the trick.
Hypothesis: You assume that if you create an ad that has a discount code, it will have a higher CTR than your existing ad, one without any discounts.
Experiment setup: You run two versions of the same ad, with the same targeting settings – the only difference is the change in the ad copy.
Ad A: This is the original ad, without any discount offer.
Ad B: Has the same copy and also features a discount offer, for example, “get 10% off everything sitewide with code: ILOVEYOGA “Shop now”.
Measurement: You track the CTR for both ads, for a specific time.
Analysis: After the A/B test, you compare the CTR of Ad A and Ad B. If Ad B has a significantly higher CTR, then well done – you’ve just confirmed that your audience responds to discount codes well.
Scenario – email marketing campaigns
For this type of A/B test, let’s imagine you run email marketing campaigns for your yoga/pilates brand. You’re looking for a way of increasing the conversion rate for your new leggings collection, and want to check which email subject line will work better. Here’s an example of A/B testing that you could run.
Hypothesis: You feel that including the client’s name in the subject line will make the email stand out, will increase the open rate, and – ultimately – the conversion rate.
Experiment setup: You take the relevant mailing list, for example, a segment of women who have shopped in the leggings category before. You split the list randomly into two segments.
Email A: Features a generic subject line (“New leggings collection available now”).
Email B: Features a personalized subject line using the recipient’s first name (“Tina, check out our new leggings collection!”).
Measurement: You establish a specific period for the experiment, for example, one week. You track open rates, click-throughs, and conversion rates for both emails.
Analysis: If email B has a higher open rate, click-through rate, and conversion rate compared to email A, it indicates that your personalization hypothesis is true. You can use this insight as a campaign optimization technique for future email marketing.
Both of these scenarios show that A/B tests let you run data-driven marketing – you have tangible results that show what type of results you can see from specific changes.
Benefits of A/B Testing
Let’s now take a quick look at why A/B testing is important.
- It helps to maximize the value of your current traffic. It’s exciting to see thousands of people visiting your site, but if this traffic doesn’t result in conversions, then it has low business value. Optimizing your website by running A/B tests will make it more appealing to visitors, resulting in more conversions.
- It helps to reduce bounce rates. You need to make your ad and website interesting enough for people to not only click on it, but also to stay long enough to find out about your offering. The more time they spend studying your page, the higher the chance they’ll convert.
- It helps with increasing conversion and boosting sales. A/B testing allows you to gain a deeper understanding of your target audience, including their goals, pain points, and preferences. You can use these insights to change certain elements in your campaign to make them more appealing to your audience, leading to more conversions, and eventually, sales.
- It helps with improving user engagement. With every new A/B test you learn more about your prospects and customers – you can see how they interact with your digital assets. This helps with visualizing user journeys and spotting roadblocks that prevent them from completing specific actions.
Examples of A/B Testing Tools
There are two general types of A/B testing tools – built-in ones from the marketing channels you use to run paid ads, and specialized A/B testing software. Here are some of the best A/B testing tools you can try.
Google Ads A/B testing tool – Drafts & Experiments
Google offers a simulation panel, which lets you test ideas for ad changes in a controlled environment, before applying them to your entire campaign.
This A/B testing tool lets you create a draft of your campaign, where you can add all the potential changes and variations such as changing your ad extensions or new keywords.
You then turn the draft into a low-risk experiment by dedicating just a small percentage of your Google Ad marketing budget to your A/B test. Google then runs your original campaign alongside your Version B. It collects performance data like clicks, conversions, and ROI for both variations. If you like the effects of the experiment, you can apply it to the whole campaign.
Facebook Ads Manager A/B testing
Similarly, Facebook lets you create different variations of the ads you’d like to test. These could include things such as changing ad settings like audience targeting, ad placement, or changes to the images and copy.
You then set your budget and add a start and end date for each variation you want to run simultaneously. Facebook automatically assigns a budget to the different variations and provides you with performance metrics both during and after the campaign.
ChannelPulse
ChannelPulse is an example of an A/B test tracking tool which brings together performance data from your paid marketing channels, including your A/B test results. It offers you a detailed analysis of what worked and what didn’t so far in your A/B tests among specific user segments.
The biggest advantage of such a tool is that you can compare results from numerous platforms. For example, if you run the same visual or messaging across TikTok and Instagram, you can see which channel brought in the best results in terms of metrics like CTR and CPA, among others.
How to Do A/B Testing
Time for practice! Here is a simple A/B testing process, which you can follow. Irrespective of what part of your campaign you want to test, you’ll have to do some preliminary work.
Take a look at your website
Before you run an A/B test, first take a deep dive into your website. Check which landing pages attract the most traffic and have the highest conversion. Also, pay attention to the type of visitors that convert. This will help you do three things: 1) pick products/services that you should focus on in your ad campaigns, 2) target the right people which should improve your ROAS, 3) direct your visitors to a site that has the highest chance of converting.
Gather information about your visitors
Deciding whom to target with your paid ads is part of a successful campaign. That’s why you should run a detailed analysis of your incoming traffic – focus on the pages that perform best. A/B testing platforms offer features like on-page surveys, heatmaps, and session recordings that will help you gather the necessary data. On top of getting information related to demographics, you’ll also gain insights into scrolling behavior, time spent on page, bounce rate, etc.
This data will help you understand the reasons for what’s stopping your visitors from performing a desired action. Fixing the issues and doing a bit of marketing optimization before starting your paid ad campaign will help you save a lot of money.
Develop a hypothesis and run A/B tests
Now you’re at a point where you know what the issues are, it’s time to make some data-backed assumptions on how to solve them. For example, imagine you run an online beauty store, and notice that a lot of visitors add products to their carts but don’t finalize the purchase. And you assume it might be a good idea to offer a discount. So you run an A/B test: version A includes a pop-up offering a 10% discount during checkout, while version B has no discount. You compare the two versions to check which one performs better, i.e., helps with reducing cart abandonment.
You can come up with a few hypotheses and test them all. Just remember to set a specific goal for your test, this can be the number of visitors, conversion rate, etc. Each test must be run simultaneously and last the same amount of time – also, split the traffic equally.
Implement the changes
This is the most important step in the A/B testing process – if your hypotheses prove right you can make the changes and focus on the winning version. However, if the results aren’t conclusive, then take a look at the data, and continue testing until you find the reason for poor ad performance. Bear in mind that A/B testing is a continuous process, rather than a task that you can simply tick off your list. Make sure to do it regularly if you want to get the best results.
A/B Testing Best Practices
Get the test hypothesis right
Sometimes, you can set your A/B testing up for failure from the start by making it about an incorrect assumption. For example, say you hypothesize that the reason why people aren’t converting from a page is due to a faulty website layout, copy, or creative. The reasons seem logical – after all, they’re common mistakes among advertisers. So, you start conceptualizing changes to see if the results will be better with the implemented changes.
However, the root cause might have nothing to do with the website itself – it can result from a technical error, like the ad redirecting to the wrong product, or sending people to their Apple Store, where they’re asked to download your app.
If you don’t check for these glitches, your A/B test results will be invalid and could lead to wrong decisions.
Test only ONE element at a time
The more hypotheses you have, the more tempting it might be to test multiple elements at a time – it’s a bad idea. While you can test all your assumptions, you need to make sure that you test one at a time.
For example, if you think that your ad might not be working because your headline and CTA are poor and you decide to test both of these elements concurrently, you won’t actually know whether the problem lies in the former or the latter. Test one element at a time to be sure you’ve identified the right issue.
Compare KPIs, and note the differences in performance for different audience groups
When you do A/B testing, it’s vital to compare key performance indicators (KPIs) and notice performance differences across various audience groups. By monitoring how click-through rates, conversion rates, and other engagement metrics vary between audience segments, you’ll be able to better tailor your strategies to meet the needs of that specific segment.
For instance, you might decide to compare two ad variants, which feature different headlines, and target two distinct groups: young adults and seniors. Version A of the ad has a heading that says:
“Get 20% Off Your Next Purchase – Limited Time Offer!”, while version B says, “Discover Our Latest Collection – Exclusive Access Inside!”. You might learn that seniors respond better to version A, while young adults to version B. Instead of picking just one winning variant, you can stick with two and simply adjust your targeting for better engagement.
Make sure that ads do not negatively influence each other
When conducting A/B testing for ads, it’s important to make sure that each ad variant is tested separately, i.e., that version A and version B aren’t shown to the same people without a change of context, so they don’t contradict each other. For example, if you’re testing two different versions of an ad, it’s important to run them against two separate groups from the same user segment or – if the hypothesis is about timing – show them individually, at different times of the day.
If there’s an overlap between your two variations, you risk the accuracy of results for each ad. Keep your ads separate – this will let you make informed decisions when it comes to what works better in terms of driving clicks, conversions, or boosting brand awareness.
Compare the same ads on different platforms
Let’s say you have a very similar audience on Facebook and Instagram in terms of demographics and price perception.
You can create two versions of an ad – their core message and goal would be the same, and they would have minor differences like platform-specific formatting.
You could launch version A on Facebook and version B on Instagram, and assign the same campaign budget to each platform. After both campaigns come to an end, you can compare the results you achieved from each channel, for the same cost.
On top of learning which ad variation worked better for the customer segment, you can also understand the differences between user behaviors and preferences on Facebook and Instagram, and adjust your future campaigns better for platform-specific experiences.
A/B Testing Meaning for Your Business
To ensure that you’re drawing the right conclusions from your A/B testing, it’s important that you don’t run them in isolation. Of course, we aren’t saying that you shouldn’t use Google’s Drafts and Experiments, or other built-in A/B testing features from the marketing platforms you use. However, we highly encourage you to test a platform like ChannelPulse that can take all the data from your A/B testing software to build a high-level picture.
ChannelPulse lets you compare your A/B experiments from numerous platforms and check how each element of an ad – be it visuals or copy – works across different platforms. This cross-referencing capability will give you more confidence when you decide what types of campaigns you should run where, and with what marketing budget.
Good luck, and here’s to getting your desired actions and conversion rates up!